XAI Bridges the Gap Between Artificial Intelligence and Human Understanding
- Prokris Group
- Dec 19, 2024
- 3 min read
Artificial intelligence (AI) is gradually becoming a systemic force shaping the modern world, driving innovation across sectors as diverse as healthcare, finance, transportation, and entertainment. Its evolution from rule-based systems to advanced machine-learning models signifies a broader technological paradigm shift, the likes of which we have only seen in critical moments of humanity’s technological evolution with the invention of steam-powered machines, electricity, computing and the internet. This type of progress always brings fundamental challenges mainly the lack of trust for new technology. There are societal concerns over bias, misuse, and disinformation, all linked to AI’s opaque architecture and a severe lack of robust governance frameworks.
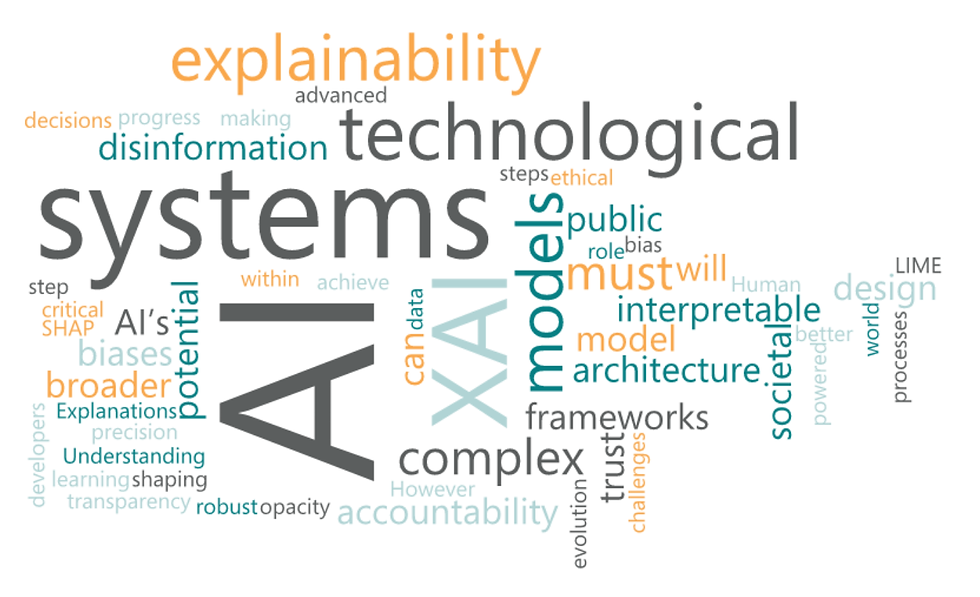
Explainable AI (XAI) emerges as a potential framework to demystify the decision-making processes of complex systems. XAI constitutes a robust technical solution required for such complex architecture and a necessary step toward bridging the gap between AI’s transformative potential and the human need for transparency, trust, and ethical accountability.
AI’s public is portrayed by big tech as a technological platform with a capacity to make decisions at scales and speeds previously unimaginable or achievable. However, the opacity of these newly developed AI-powered processes raises critical questions in relation to the profit-driven agenda of the developers of such systems. Explainable AI could address this lack of opacity by making the core architectural elements of AI systems interpretable and accessible. Techniques such as LIME (Local Interpretable Model-agnostic Explanations) and SHAP (SHapley Additive exPlanations) demonstrate progress in understanding better the way certain AI systems are built and behave.
For instance, LIME simplifies complex predictions by creating interpretable models tailored to individual instances, while SHAP quantifies feature contributions to model decisions. These methodologies could transform AI systems and the companies that develop them and operate them into more transparent partners. XAI could empower users to enhance the quality of datasets, modify algorithms, and achieve superior results simply by streamlining the explainability and visibility of errors and biases.
Although the potential is considerable, achieving explainability is a complex undertaking. Advanced models, particularly in deep learning, are often impenetrable even to experts. The challenge is to balance that level of interpretability with accuracy and consistency. Simplified models risk compromising precision, while highly accurate systems resist simplification and explainability. This is by design, as everyone is entangled in the race of who will introduce the latest, faster, better model, which renders this level of accountability and responsibility impossible to achieve. We must keep in mind that XAI systems are not immune to biases embedded within data or design.
However, it is a step towards more rigorous scrutiny of AI system development and our effort to design explainability frameworks that prioritise fairness, precision, and inclusivity.
XAI’s architecture can be instrumental in addressing broader societal concerns about AI. The malicious use of AI, from disinformation campaigns to automated hacking, showcases the risks inherent in unchecked technological advancement.
Studies have evidenced bias in AI systems. Unfortunately, models trained on biased data perpetuate inequities, leading to discriminatory outcomes. XAI can play a role here, exposing these biases and forcing corrective action. AI-generated disinformation threatens the integrity of public discourse. The challenges AI presents extend beyond explainability alone. It is imperative that we mitigate this by employing and implementing additional steps such as improving media literacy and technological safeguards and enforcing greater accountability from developers.
Big tech must embrace transparency, governments must establish enforceable regulations, and the public must be equipped with the knowledge to engage critically with AI systems. Explainable AI (XAI) could serve as a foundational element in these steps, yet its efficacy will depend on broader collaboration within approved ethical frameworks.
In this period of societal transformation, explainable AI (XAI) can play a crucial role for the responsible development and use of artificial intelligence. Its efficacy will be determined by technological advancements and by its capacity to enable humanity to comprehend, trust, and influence the technologies that are progressively shaping our world.
Comments